INFORMS 2021 Practice Talks
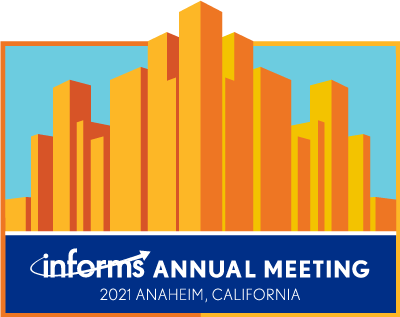
INFORMS Practice Session with Pittsburgh Professionals
The 2021 INFORMS annual conference approaches, and many students from CMU will be attending to present their work!
In collaboration with the Pittsburgh INFORMS Professional Chapter, we held a series of practice talks where students had the opportunity present their talks to fellow researchers and industry professionals. We had some great feedback on how to sell one’s work to different audiences and how to tell an engaging story through the talk.
We hope to collaborate with the Pittsburgh Professional Chapter through other events in the future. We feel they could provide valuable insights into the applications of theoretical work that is sometimes neglected in academic settings.
Many thanks our fantastic speakers:
-
Victoria Achkar - Integrated Guaranteed Service Approach for Multi-echelon Inventory Optimization
Abstract: The aim of the Guaranteed Service Model is to allocate safety stocks across the network so as to reach target service levels at the lowest cost, accounting for demand uncertainty. The main contribution of this work is the development of an extended and integrated approach that accounts for a multi-product supply chain with stochastic demand and lead times, review periods, minimum order quantities, hybrid nodes, and fill rates as an alternative measure to set safety stocks. We propose a QCP reformulation and evaluate their performance by solving a real case study. -
Aaron Burns - Smart Curb Optimization Increases Energy Productivity of Delivery Vehicle Operations
Abstract: When loading zones reach capacity, delivery vehicles must double-park or cruise in search of parking, increasing energy consumption and externalities via additional delivery vehicle travel and road congestion effects. Smart curbs that manage and assign loading zone parking demand have the potential to coordinate delivery vehicles and available parking spaces to reduce energy consumption. We adapt and apply a mixed integer linear programming parking slot assignment formulation to optimize smart curb parking space assignment to delivery vehicles to minimize double parking. Across scenarios ranging from 1 to 200 delivery vehicles per day and 1 to 4 spaces, we find that smart curb optimization can, on average across our cases, reduce double parking by 20 minutes to multiple hours given an increasing number of parking spaces. We also propose a queuing model for future use in our research to better estimate the energy use and externalities associated with double parked delivery vehicles. -
Thomas Lavastida - Robust Machine Learned Predictions For Online Allocation
Abstract: This talk considers a beyond-worst-case analysis model that integrates machine learned predictions into algorithm design. We give an end-to-end framework for incorporating predictions into online allocation problems including Adwords, matching, flow allocation, and scheduling. Our algorithms give near optimal performance with good predictions. Moreover, they are robust to prediction error and the quality degrades gracefully in terms of the error. Empirical results demonstrating the quality of the algorithms as well as the learnability of the predictions validate our theoretical findings. -
Mik Zlatin - On Small-Depth Tree Augmentations
Abstract: In the Weighted Tree Augmentation Problem (WTAP), we are given an undirected tree, and an additional set of links with non-negative costs. The objective is to find the minimum cost subset of links to add to the tree so that the resulting network is 2-edge-connected. We show that the integrality gap of the ODD-LP relaxation for WTAP on a k-depth tree instance is at most \(2-1/2^k\) . For 2- and 3-level trees, these ratios are 3/2 and 7/4 respectively. Our proofs are constructive and yield polynomial-time approximation algorithms with matching guarantees.